Unlocking Opportunities: The Role of Data in Medical Machine Learning
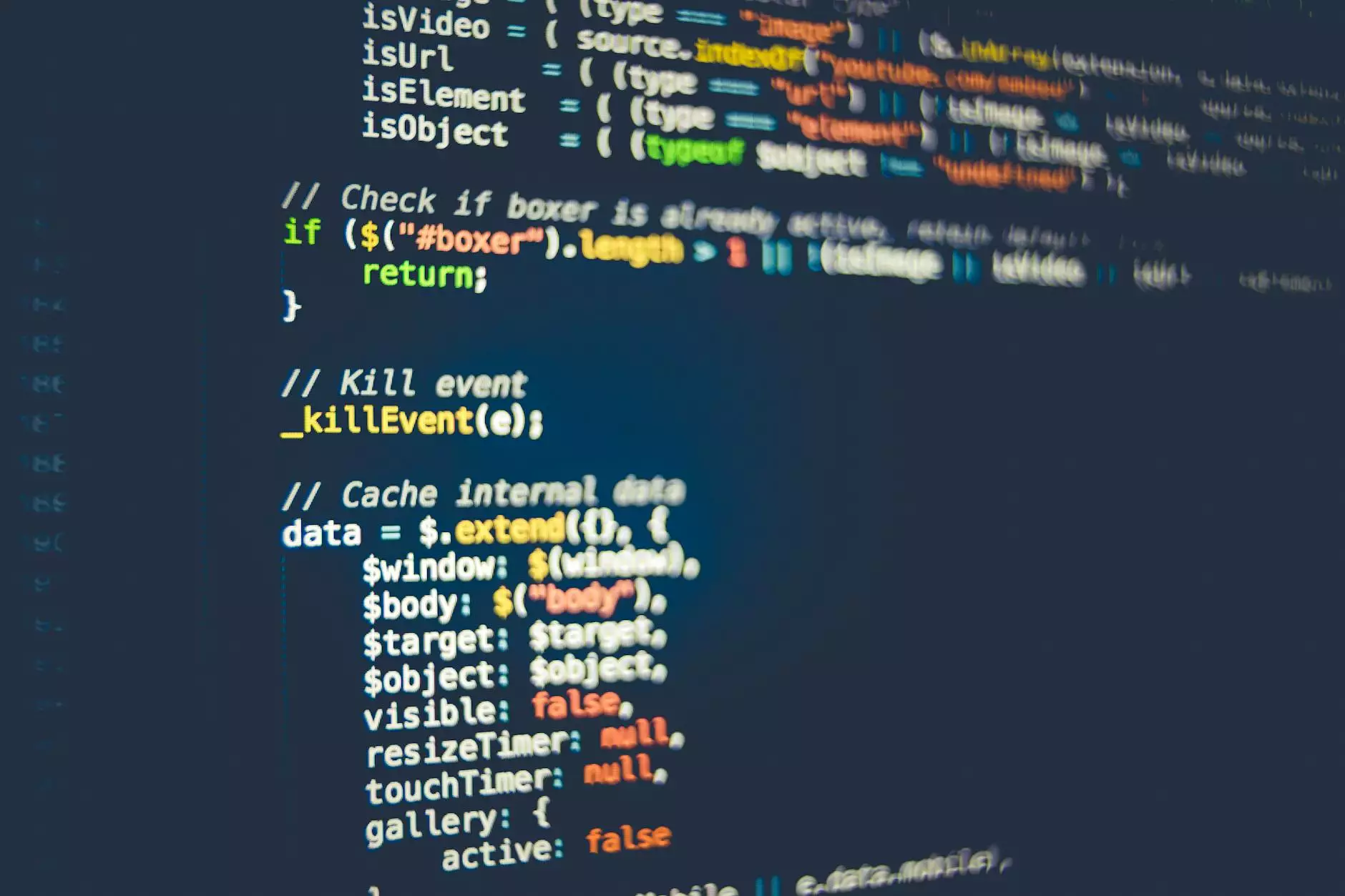
The dawn of the digital age has transformed numerous industries, with healthcare being one of the most profoundly affected. At the heart of this transformation lies the power of data. In the realm of machine learning, medical datasets have emerged as critical tools that fuel innovations not just in clinical settings, but also in administrative and operational capacities.
The Importance of Medical Datasets in Machine Learning
Machine learning represents a revolutionary approach to data analysis, enabling computers to learn from datasets without being explicitly programmed. In the medical field, this means utilizing medical datasets for machine learning to develop predictive models, enhance decision-making, and improve patient outcomes remarkably.
Understanding What Constitutes a Medical Dataset
A medical dataset typically consists of an organized collection of data that includes various aspects of patient health, clinical trials, and healthcare outcomes. Key elements in these datasets may include:
- Patient Demographics: Age, gender, ethnicity, and socioeconomic status.
- Medical History: Previous diagnoses, treatments administered, and outcomes.
- Clinical Measurements: Vital signs, laboratory test results, and imaging data.
- Treatment Details: Types of interventions, dosages, and durations.
- Outcome Data: Recovery rates, patient satisfaction surveys, and readmission statistics.
How Medical Datasets Propel Machine Learning Innovations
The integration of machine learning technologies with comprehensive medical datasets revolutionizes several facets of healthcare, including:
1. Predictive Analytics
One of the most significant applications of medical datasets in machine learning is in predictive analytics. By analyzing historical patient data, healthcare providers can identify trends and predict future outcomes. For example:
- Risk Assessment: Predicting the likelihood of diseases such as diabetes or cardiovascular issues.
- Hospital Readmission: Determining which patients are at greater risk of returning after discharge based on previous admissions.
2. Personalized Medicine
Personalized medicine aims to tailor treatment plans to individual patients based on genetic, environmental, and lifestyle factors. Medical datasets for machine learning enable the development of models that can match patients with the most effective therapies, ultimately improving outcomes and minimizing adverse effects.
3. Drug Development
Machine learning methods applied to medical datasets can expedite the drug discovery process significantly. By analyzing the effectiveness of compounds during clinical trials, researchers can:
- Identify Potential Candidates: Quickly narrow down promising medications based on patient response data.
- Reduce Costs: Minimize the financial burden associated with failed drug trials.
Challenges and Ethical Considerations
While the potential of medical datasets and machine learning is immense, there are crucial challenges that the industry must address, including:
Data Privacy and Security
With the immense power of data comes the responsibility to protect patient information. Compliance with regulations like HIPAA in the U.S. is essential to safeguard sensitive health information while utilizing datasets for research and analysis.
Bias in Data
Bias in medical datasets can lead to skewed results and inequitable care. Ensuring diversity in datasets is vital for building models that accurately reflect and cater to varied populations.
Building Comprehensive Medical Datasets
Creating a robust medical dataset is a daunting task, requiring meticulous planning and execution. Healthcare organizations must ensure that:
Data Collection is Systematic
Establishing standardized protocols for data collection helps guarantee accuracy and reliability. This includes:
- Consistent Terminology: Utilizing standardized medical terminology to avoid ambiguity.
- Regular Updates: Keeping datasets current to reflect the latest medical findings and patient demographics.
Data Integration
It’s essential to integrate data from various sources, including clinical workflows, patient records, and wearable health technologies. This integration facilitates a more holistic understanding of patient health.
The Future of Medical Machine Learning
The future of healthcare promises to be influenced heavily by advancements in machine learning and medical datasets. As technology advances, we can expect to see:
Improved Patient Outcomes
Through continuous learning and improvement, machine learning models can help healthcare professionals make better, data-driven decisions that enhance patient care.
Greater Efficiency in Clinical Operations
By automating routine tasks and information processing, machine learning can allow healthcare providers to focus on what matters most—caring for patients.
Enhanced Research and Development
The field of medical research will benefit from the ability to analyze large datasets quickly, leading to faster discoveries and innovations in treatment options.
Conclusion
The intersection of medical datasets for machine learning represents a frontier of possibilities that can redefine how healthcare is delivered and appreciated. As stakeholders in the healthcare ecosystem embrace these technologies, they are poised to unlock solutions to some of the most pressing challenges facing the industry today.
Healthcare organizations must commit to investing in technology, adhering to ethical practices, and ensuring the integrity of their datasets. This commitment will pave the way for a safer, more efficient healthcare system that ultimately benefits everyone involved.
Together, as we continue to harness the potential of machine learning and comprehensive medical datasets, we will undoubtedly unlock new opportunities and innovate the keys to improving health outcomes on a global scale.
medical dataset for machine learning