The Transformative Impact of Machine Learning Annotation Tools in Software Development
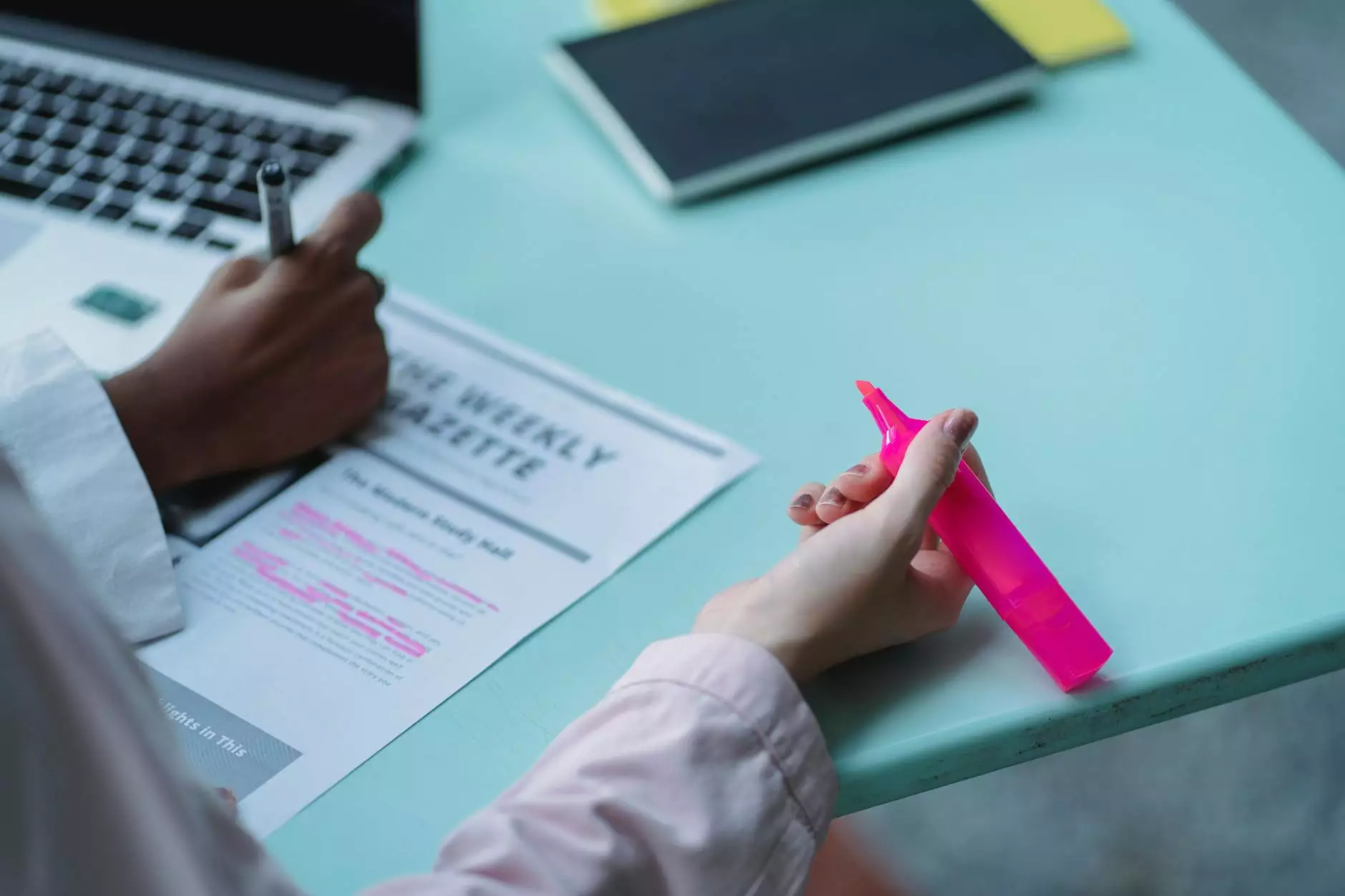
Software development is at the forefront of a technological revolution, particularly with the rise of artificial intelligence (AI) and machine learning (ML). A pivotal aspect of this revolution entails the processing and understanding of vast amounts of data. To offer enhanced performance and accuracy, machine learning models require high-quality data, meticulously prepared and annotated. This is where machine learning annotation tools come into play, becoming vital components in the software development lifecycle.
Understanding Machine Learning Annotation Tools
Machine learning annotation tools are software applications designed to assist in labeling data, a crucial step in training ML algorithms. By annotating data sets, these tools help in creating structured data that ML models can learn from, ultimately improving their predictive accuracy and effectiveness in real-world applications.
What Are Data Annotations?
Data annotation refers to the process of labeling or tagging the data to provide context. This context is essential for machine learning algorithms to understand and learn patterns. Various types of data require specific annotation techniques:
- Image Annotation: Tagging objects or features within images (e.g., identifying animals in wildlife photography).
- Text Annotation: Marking specific parts of text, identifying entities or sentiments (e.g., classifying customer reviews).
- Audio Annotation: Labeling speech segments and sounds in audio files (e.g., distinguishing between background chatter and a speaker).
- Video Annotation: Identifying critical events or objects within video clips (e.g., detecting player movements in sports footage).
The Significance of High-Quality Annotations
For machine learning models, the quality of annotations directly impacts the model's performance. High-quality annotations lead to:Improved Accuracy: Well-labeled data enables models to learn more effectively, which enhances prediction accuracy.Reduced Training Time: With clear and precise labels, models need less time to learn, speeding up project timelines.Better Generalization: High-quality data leads to improved model performance in unseen data, a critical aspect of machine learning.
Challenges in Data Annotation
While the importance of machine learning annotation tools cannot be overstated, various challenges exist:
- Scalability: As data volume grows, manually annotating data becomes impractical. Automated tools provide solutions.
- Consistency: Variability in human annotation can lead to inconsistent data quality. Automated solutions can standardize the process.
- Subjectivity: Ambiguity in data requires clear guidelines to ensure that annotations remain objective and accurate.
Benefits of Using Machine Learning Annotation Tools
1. Enhanced Efficiency
With the help of advanced algorithms, machine learning annotation tools can significantly accelerate the annotation process, allowing teams to handle larger datasets in a shorter amount of time. Automation reduces the dependency on manual inputs, freeing up valuable human resources for more complex tasks.
2. Cost-Effectiveness
Although investing in machine learning annotation tools may seem costly initially, they yield long-term savings. By reducing labor costs and minimizing errors, these tools can lead to a higher return on investment (ROI) for organizations pushing forward with their AI projects.
3. Collaboration and Integration
Many annotation tools are designed to integrate seamlessly with existing software development environments and collaboration platforms. This integration allows teams to collaborate on data annotation in real-time, ensuring that everyone remains aligned with project objectives and deadlines.
Choosing the Right Machine Learning Annotation Tools
Selecting the ideal machine learning annotation tool can be overwhelming given the plethora of options available. Consider the following features to make an informed choice:
- User-Friendly Interface: A tool that offers ease of use can significantly boost productivity.
- Customizable Workflows: The ability to tailor workflows to fit specific project requirements enhances flexibility.
- Support for Multiple Data Types: Choose tools that can handle various types of data, including images, videos, text, and audio.
- Quality Control Mechanisms: Features that ensure consistency and accuracy in annotations are vital.
- Scalability: As projects grow, the tool should accommodate increased data without compromising performance.
Leading Machine Learning Annotation Tools on the Market
1. Labelbox
Labelbox is a powerful, user-friendly tool for image annotation. It provides robust collaboration features and supports various data formats, making it a favorite among software developers.
2. Amazon SageMaker Ground Truth
Amazon SageMaker Ground Truth is designed to streamline the annotation process with built-in machine learning functionalities. It offers manual and automated data annotation options, catering to diverse needs.
3. Supervisely
Supervisely combines flexibility with a vast array of tools for video and image annotation, allowing teams to ~focus on creating high-quality datasets efficiently.
4. V7 Labs
V7 Labs offers powerful image and video annotation tools. With features like pre-annotation and smart suggestions, it minimizes time consumption and maximizes accuracy.
Real-World Applications of Annotated Data
Annotated data is the backbone of numerous AI applications, enabling advancements in various sectors:
- Healthcare: Data annotation aids in medical imaging, enabling AI models to detect anomalies in scans.
- Autonomous Vehicles: Labelled data is crucial for training models that interpret sensor data and navigate safely.
- Retail: Consumer behavior analysis through annotated data helps businesses tailor marketing strategies and improve customer experiences.
- Natural Language Processing: Text annotations enhance model understanding of context, leading to better sentiment analysis and language translation.
Future Trends in Machine Learning Annotation
As AI technologies continue to advance, the demand for machine learning annotation tools will grow. Some predicted trends include:
1. Increased Automation
The future of machine learning annotation lies in automation. Advanced algorithms will minimize manual efforts while maximizing annotation quality.
2. Intelligent Annotation
Enhanced AI capabilities will lead to intelligent annotation tools capable of auto-labeling data with greater accuracy, significantly streamlining the process.
3. Integration with ML Models
In the near future, annotation tools may offer seamless integration with machine learning models, enabling real-time learning and adaptation.
Conclusion
The integration of machine learning annotation tools in software development is critical for harnessing the full potential of AI. As the industry continues to evolve, organizations that adopt these tools will gain a competitive edge, allowing them to enhance their efficiency, improve data quality, and ultimately, deliver superior products and services.
In a world increasingly driven by data, the ability to annotate accurately and efficiently will define the success of machine learning initiatives. By investing in the right tools and understanding their capabilities, businesses can pave the path towards innovative solutions and groundbreaking advancements.