The Transformative Power of Video Labeling Tools in Machine Learning
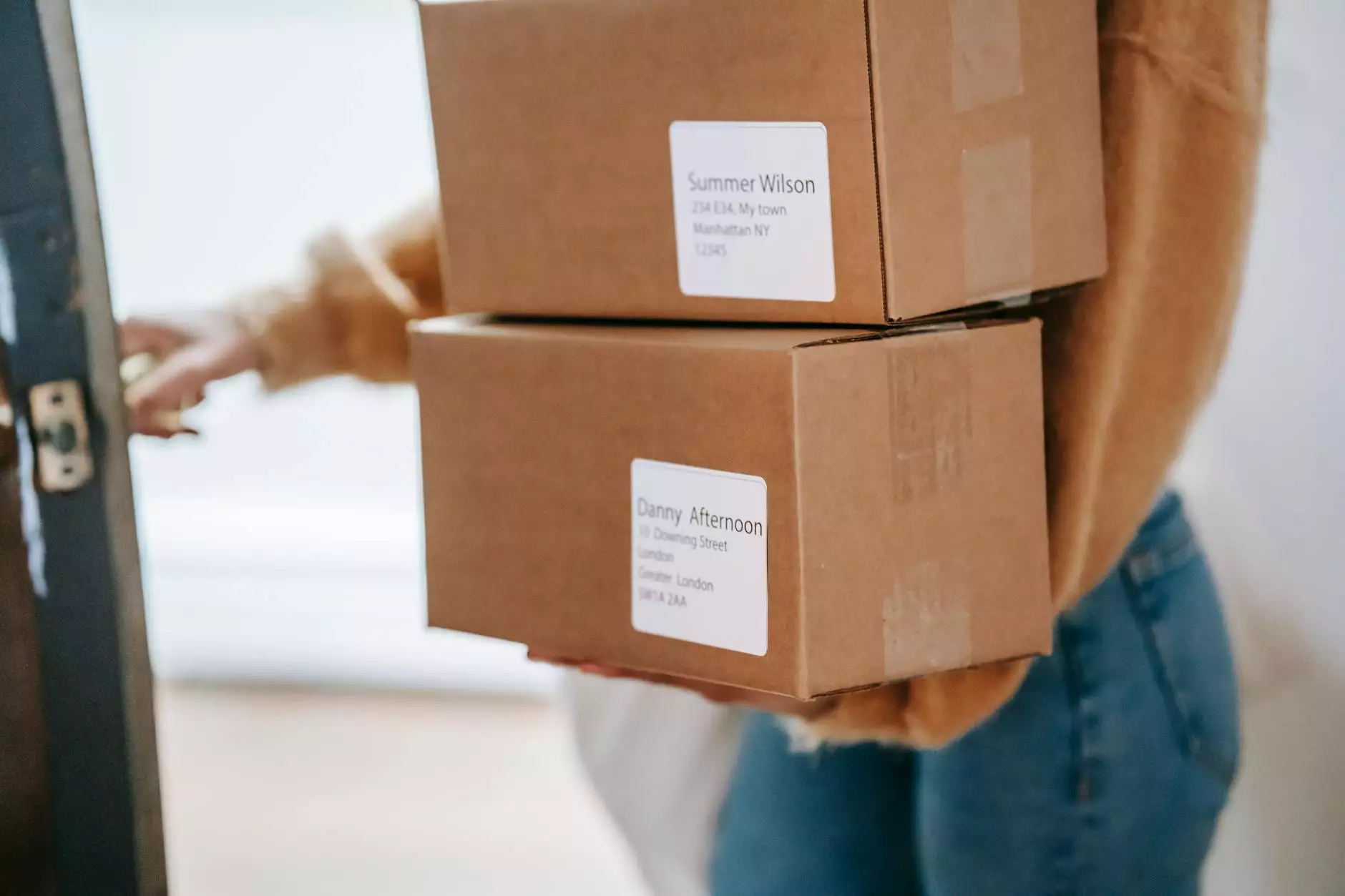
In today’s fast-paced digital environment, where data is considered the new oil, the significance of tools that can enhance the efficiency of machine learning (ML) is undeniably vital. One of the most revolutionary advancements in this arena is the introduction of the video labeling tool for machine learning applications. This article delves deep into how these tools function, their benefits, and the impactful role they play in data annotation.
Understanding the Basics of Video Labeling Tools
At its core, a video labeling tool is a software application designed to assist in the process of annotating and labeling videos for machine learning models. This process is essential as it provides the ground truth data that ML algorithms require to learn effectively. Without accurately labeled data, the performance of ML models can drastically decline.
Key Features of Video Labeling Tools
- User-Friendly Interface: Most video labeling tools include intuitive interfaces that allow users to easily navigate through complex labeling tasks.
- Multiple Annotation Types: They support various annotation methods, such as bounding boxes, semantic segmentation, and key point annotations, tailored for diverse ML needs.
- Collaboration Capabilities: Many tools offer features for team collaboration, enabling multiple users to work on the same project simultaneously.
- Integration with ML frameworks: Seamless integration with popular machine learning libraries and frameworks to streamline workflows.
The Importance of Data Annotation in Machine Learning
Data annotation is often regarded as the backbone of machine learning. Without clean, labeled data, even the most sophisticated algorithms struggle to perform efficiently. Annotating video data, in particular, presents unique challenges.
Challenges in Video Data Annotation
Video data annotation involves temporal and spatial complexities. Unlike static images, videos require analysis over time, which can be resource-intensive. The challenges include:
- Dynamic Environments: Videos often contain moving objects, necessitating frame-by-frame analysis.
- Context Awareness: The context in which an object appears may change over time, complicating correct labeling.
- Volume of Data: Given the continuous nature of video, the sheer volume of data can overwhelm traditional annotation methods.
How Video Labeling Tools Address These Challenges
The advent of video labeling tools has greatly alleviated the burden of video data annotation. Below are several ways in which these tools make the process more efficient:
Automated Annotation Features
Some advanced video labeling tools leverage machine learning algorithms to automatically annotate portions of the video, significantly speeding up the process. This is particularly useful when dealing with well-defined objects and environments.
Efficient Workflow Management
Many video labeling tools offer project management functionalities that allow for tracking progress, collaboration, and resource allocation. This ensures that teams can handle larger projects more effectively.
Adaptive Learning Over Time
With features that enable feedback loops, video labeling tools can learn from user inputs over time, improving the quality of automated annotations and making the process faster and more reliable.
Applications of Video Labeling Tools in Machine Learning
The potential applications of video labeling tools in machine learning are vast. Here are some industries where they have made a significant impact:
1. Autonomous Vehicles
In the automotive industry, video labeling tools are crucial for training self-driving car systems. They allow for precise mapping of road conditions, pedestrian movement, and traffic signals, which are imperative for the safety and functionality of ML systems in vehicles.
2. Surveillance and Security
Video surveillance systems utilize ML for threat detection and anomaly recognition. Accurate annotations in the training data help in identifying suspicious activities, enhancing public safety measures.
3. Sports Analytics
In sports, analyzing video footage through machine learning can provide insights into player performance and strategy. Labeling game footage helps in developing models that assess player movements and plays effectively.
4. Healthcare and Medical Imaging
In the healthcare sector, video labeling tools assist in analyzing patient behavior and movements, which can be crucial for rehabilitation programs and patient monitoring.
Choosing the Right Video Labeling Tool
With countless video labeling tools available, selecting the right one can be overwhelming. Here are some critical factors to consider:
1. Scalability
Choose a tool that can grow with your business and scale according to the volume of data you handle.
2. Customization Options
The tool should offer customization to cater to specific annotation needs and workflows.
3. Support and Community
A strong support system and active user community can significantly enhance your experience, providing assistance and sharing best practices.
4. Cost-Effectiveness
Evaluate the pricing structure of the tool to ensure it aligns with your budget while meeting your operational requirements.
Future Trends in Video Labeling Tools and Machine Learning
The future of video labeling tools and machine learning is brimming with possibilities. As technology advances, here are some trends to watch:
1. Increased Automation
We can expect a trend toward further automation in video labeling, as AI and ML algorithms improve their accuracy and efficiency in annotation tasks.
2. Enhanced Real-Time Processing
Real-time video processing capabilities will become more commonplace, allowing for immediate feedback and rapid model updates based on new data.
3. Cross-Platform Integration
Tools will increasingly integrate with various data platforms and machine learning frameworks, creating seamless workflows from data acquisition to analysis.
4. Ethical AI Practices
As awareness of ethical AI practices grows, video labeling tools will also incorporate guidelines to ensure that data used for training does not embed biases and respects privacy.
Conclusion: The Impact of Video Labeling Tools
In conclusion, the significance of video labeling tools in machine learning cannot be overstated. They are essential for ensuring accurate data annotation, ultimately leading to more robust and reliable machine learning models. Organizations aiming to harness the full potential of their data must invest in quality annotation tools that adapt to the rapidly evolving landscape of machine learning technologies.
For those interested in leveraging cutting-edge data annotation solutions, KeyLabs.ai offers a range of advanced tools designed for both ease of use and effectiveness. Explore how you can elevate your projects today!
video labeling tool machine learning